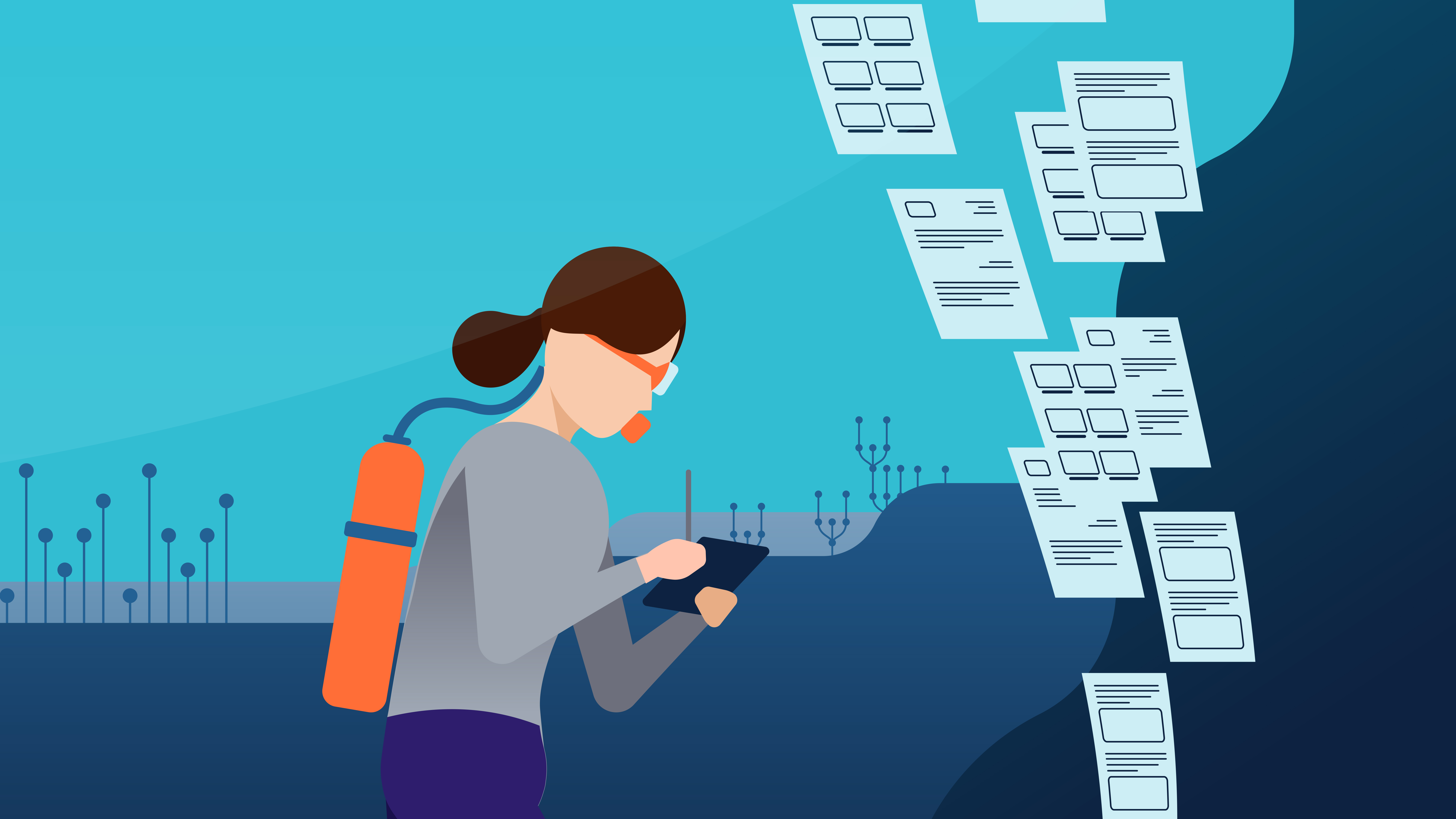
Everyone’s talking AI but do they really understand it? Getting AI to work for your business doesn’t have to be daunting. Here at Elula we help businesses cut through the myths and clear the path, delivering tangible ROI and helping retain customers. Here are the top 10 AI myths we hear all the time and the truth behind them.
1. “I can’t do AI because I haven’t centralised my data yet.”
This is a common view held. Most organisations do have messy, disparate data and we know how difficult it can be to access and interpret this especially with limited resources and competing priorities. Our approach is to do all the hard work for you, quickly bringing your datasets together in one place. We centralise, cleanse, transform and engineer the data for you ready for use in our software products which helps you focus on the key business problems you need to solve – retaining and engaging customers.
2. “Non-technical people can never understand AI.”
Like all deep technical skill sets, with the right people explaining it, AI becomes a lot easier to grasp. At Elula we translate all the technical jargon into everyday business context, so you don’t have to be a tech guru to understand how it comes together. We’ve designed our products for the front line and show you how AI can help you engage with your customers in a more meaningful way. This is not just boasting; it’s a distinguishing feature of our product versus others.
3. “AI is plug and play. Just download a software package, run your data through it and off you go.”
Nope. Delivering productionised AI is a complex task with a number of pitfalls to be wary of. If you try this approach, you’ll make many mistakes. Either the product you expected to work, won’t work or you’ll think it works when in fact it doesn’t. AI isn’t as simple as ‘plug and play’, it requires a bespoke and integrated approach to be effective. Remember, AI is not one thing, it’s made up of many different models and it’s only effective when you put the right algorithms to work on the right problem. There’s a reason that the vast majority of AI projects fail and it’s often from overlooking the most critical component – having a deep understanding of the business problem being solved.
4. “Data is all you need.”
Data alone won’t get you there. AI requires real domain and business knowledge combined with deep technical expertise. Without this, you would simply be diving into a prediction model with no understanding of the industry you are operating in. Let’s look at the banking sector for example. To make sense of a bank customer, you need to understand how a bank works, what a mortgage is and why a person even has a mortgage in the first place. This real-world knowledge is what leads to commercial value. If it were that easy, companies would just automatically turn data into insights – just like buying a bag of magic beans.
5. “I just need to hire a Data Scientist to sort it all out for me.”
If only it were that simple. There are multiple parts to AI, data scientists being one part along with machine learning engineers and data engineers. Each have specific skill sets across different disciplines required to make an AI initiative successful. But even within the data science discipline there are a multitude of skillsets each dependent on the business problem you’re solving. If you want to play Beethoven you don’t hire an electric guitarist just because they’re a musician! The same is true of data science. It’s not simply a matter of hiring someone, it’s having the correct team in place for each task.
6. “When the model is built, the project is finished.”
Data science and setting up the initial modelling is just one part of it. Execution and dedicated on-going maintenance is the rest and in fact, where the actual value is realised. What most organisations overlook is implementing data science models into production and then rollout with the users. It is critical to make sure that the end-user can take action and gain value from the outputs.
7. “I can reskill my existing team to become Data Scientists.”
Sure, you could sign your existing team up to a crash course in AI. In reality, most courses are of little value without real world experience and a reasonable knowledge of data science requires years of study. A question to consider is how much do you really want a novice experimenting with your organisation? What’s more, good Data Scientists also need to have the right problem-solving mindset, and if they’re not the right kind of analytical mathematical thinker, no course is ever going to fix that.
8. “AI decisions come from mysterious black boxes.”
For the majority of solutions out there this is true but there is a way to understand machine learning model outputs and discover why AI decisions are made. At Elula, we use our proprietary explainable AI to unpack the black box mystery. Our industry leading approach to explainable AI provides tangible reasons for every action, providing the reasons a prediction model has arrived at an outcome for each individual customer. We’ve written on this in the past and you can find out more here.
9. “We’re too small for AI.”
At Elula, we work with all sorts of companies who use our AI software to help them better understand their customers. Our products deliver actions and outcomes that focus right in on the customers you need to speak to and it works equally well across both small and large organisations.
10. “All Data Scientists play Dungeons and Dragons.”
Incorrect. 25.593454533% do. The rest play Pentago.